Data Analysis: How to Properly Choose Statistical Tests?
- Karolina Dyrla-Mularczyk
- Aug 19, 2024
- 4 min read
Updated: Mar 17
If you are writing your master’s or doctoral thesis or conducting scientific research, you may be faced with the challenge of selecting the appropriate tools for data analysis. This choice is crucial, as selecting the correct statistical test helps avoid errors in reasoning, and precise statistical analysis allows for drawing accurate and reliable conclusions. When choosing a statistical test, several factors must be considered: the type of variables, the number of groups being studied, data distribution, dependency of samples, and sample size.
1. Type of Variables:
Nominal: If dealing with data such as gender (a dichotomous nominal variable), eye color, or country of origin, you would use the chi-square test or Fisher’s exact test. For dichotomous nominal variables, the binomial test might also be a good choice.
Ordinal: If analyzing variables such as education level or satisfaction level (categorical variables with a certain order or hierarchy), rank-based tests like the Mann-Whitney U test are suitable. It’s important to note that the Mann-Whitney U test is also used for quantitative data and is non-parametric, meaning the data distribution does not need to follow a normal distribution. It compares medians between two independent groups (not the mean).
Quantitative: When analyzing data expressed on a ratio or interval scale, such as raw psychological test scores, time, income, or blood glucose levels, parametric tests (e.g., t-tests) or non-parametric tests (e.g., the Wilcoxon test) are used.
2. Number of Groups Studied:
Two Groups: When analyzing results from two groups, you would use an independent or paired t-test, or the non-parametric equivalent – the Mann-Whitney U test.
More Than Two Groups: In this case, you would use tests like ANOVA or the Kruskal-Wallis test (when comparing medians and the data do not meet the assumptions of normal distribution and homogeneity of variance).
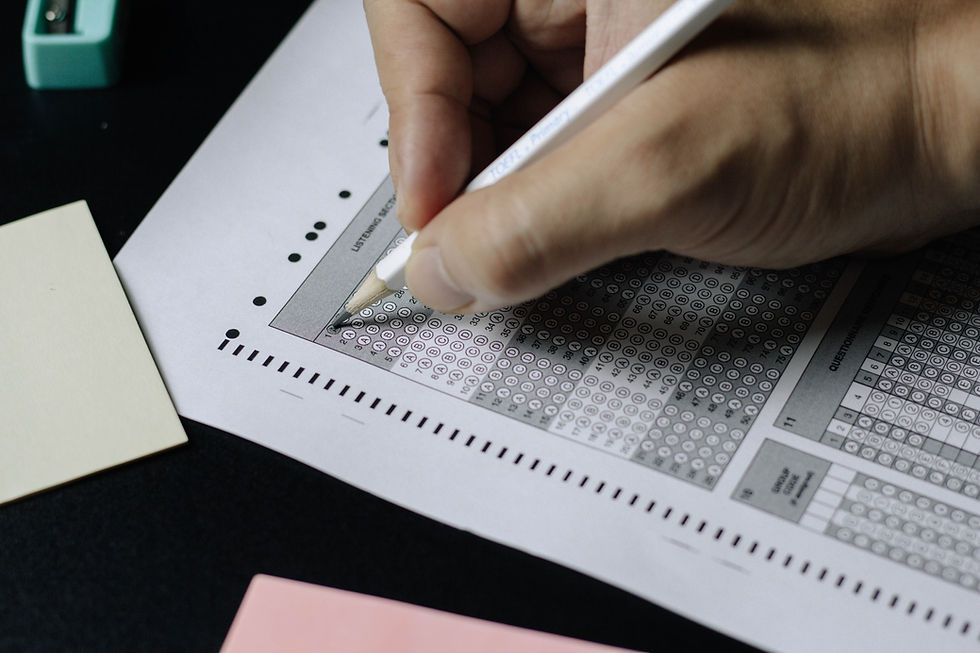
3. Data Distribution:
If the data meet assumptions of normality, homogeneity of variance, independence of observations, and randomness of the sample, parametric tests (such as the Student’s t-test or ANOVA) are chosen.
If the assumptions are not met, non-parametric tests like the Mann-Whitney U test or Kruskal-Wallis test are used. However, remember the limitations of these tests! One limitation is lower statistical power, meaning these tests are less capable of detecting actual effects (differences between groups). This may lead to a higher risk of a Type II error (false acceptance of the null hypothesis). Non-parametric tests typically do not provide estimates of population parameters (e.g., mean or variance), limiting the range of information that can be obtained about the studied population. The results of non-parametric tests can also be more challenging to interpret, particularly regarding effect size.
Mann-Whitney Test and Its Limitations
The Mann-Whitney test does not directly compare medians, although it is often interpreted that way. Its main purpose is to assess whether the values in one group tend to be larger (or smaller) than those in another group, indicating differences in distributions. Therefore, it is important to keep in mind some key limitations of this test:
If distributions differ in shape (e.g., one group has greater variance), the test may detect a difference even when the medians are equal.
If the goal is a strict median comparison, a better approach might be the Hodges-Lehmann estimator for the median difference or other confidence interval-based methods.
4. Dependency of Samples:
If dealing with independent samples—where the measurements in one group are entirely independent of those in another group—you would choose an independent t-test or ANOVA (for more than two samples) or the non-parametric Mann-Whitney U test.
If dealing with repeated measures or paired samples, a paired t-test, ANOVA (for more than two samples), or the non-parametric equivalent—the Wilcoxon signed-rank test—is appropriate.
5. Sample Size:
For small samples, non-parametric tests are preferred.
For large samples, parametric tests are chosen, provided the assumptions are met.
A small sample is generally considered to be up to 30 observations. This approach is based on the Central Limit Theorem, which states that the distribution of the sample mean will approach a normal distribution as the sample size increases, regardless of the population distribution. In practice, a sample size of 30 is considered sufficient for this effect to be noticeable. For samples larger than 30, the distribution of the sample mean is usually sufficiently close to a normal distribution.
When Can the Central Limit Theorem (CLT) Be Misinterpreted?
🔹 The "magic number 30" misconception – It is often said that a sample size "greater than 30" is sufficient for the distribution of means to be normal. However, if the population is highly skewed or contains outliers, a much larger sample may be needed. In extreme cases (e.g., distributions with infinite variance), CLT may not even hold.
🔹 It does not apply to a single sample! – CLT describes the distribution of means across multiple samples, not the shape of an individual sample of data. If you have only one sample and want to check its normality, you must do so empirically rather than relying on CLT.
🔹 Not all statistics follow a normal distribution – CLT applies to sample means, but not necessarily to other statistics (e.g., variance, skewness).
It’s important to remember that applying the correct statistical tests ensures high standards in data analysis and helps draw accurate conclusions. It also helps avoid errors and increases the credibility of your research.
If data analysis for your master’s or doctoral thesis is keeping you up at night, or if statistical analysis still feels like black magic to you, feel free to contact us!